Generative AI and large language models (LLMs) have opened new avenues for innovation and understanding across the digital domain. This became possible because LLMs trained on massive internet text datasets can learn the fundamental structure of language. What if AI models could also learn the fundamental structure of physical behaviors–how the physical world around us changes over space and time? What if AI could help us understand the behaviors of real world systems, objects, and people?
At Archetype AI, we believe that this understanding could help to solve humanity’s most important problems. That is why we are building a new type of AI: physical AI, the fusion of artificial intelligence with real world sensor data, enabling real time perception, understanding, and reasoning about the physical world. Our vision is to encode the entire physical world, capturing the fundamental structures and hidden patterns of physical behaviors.
We’re building the first AI foundation model that learns about the physical world directly from sensor data, with the goal of helping humanity understand the complex behavior patterns of the world around us all. We call it a Large Behavior Model, or LBM. Here’s how it works.
Getting Data From The Real World
LLMs get their data from text—the vast corpus of writing humans have added to the internet over the last several decades. But that is the extent of their knowledge.
The web captures just a small glimpse of the true nature of the physical world. To truly understand real world behaviors, an AI model must be able to interpret much more than human-friendly data like text and images. Many physical phenomena are beyond direct human perception: too fast-moving, complex, or simply outside the realm of what our biological senses can detect. For example, we can not see or hear the temperature of objects or the chemical composition of air. An AI model trained only on a human interpretation of the physical world is inherently limited and biased in its understanding.
Our foundation model learns about the physical world directly from sensor data – any and all sensor data. Not just cameras and microphones, but temperature sensors, inertial sensors, radar, LIDAR, infrared, pressure sensors, chemical and environmental sensors, and more. We are surrounded by sensors that capture rich spatial, temporal, and material properties of the world.
Different sensors measure different physical properties and phenomena which are related and correlated. To encode the entire physical world, information from all these sensors must be encoded, fused and processed into a single representation. This fusion enables a holistic approach in perceiving and comprehending the physical world. And by allowing the model to ingest sensor data directly from the physical world, we can have it learn about the world without human biases or perceptive limitations.
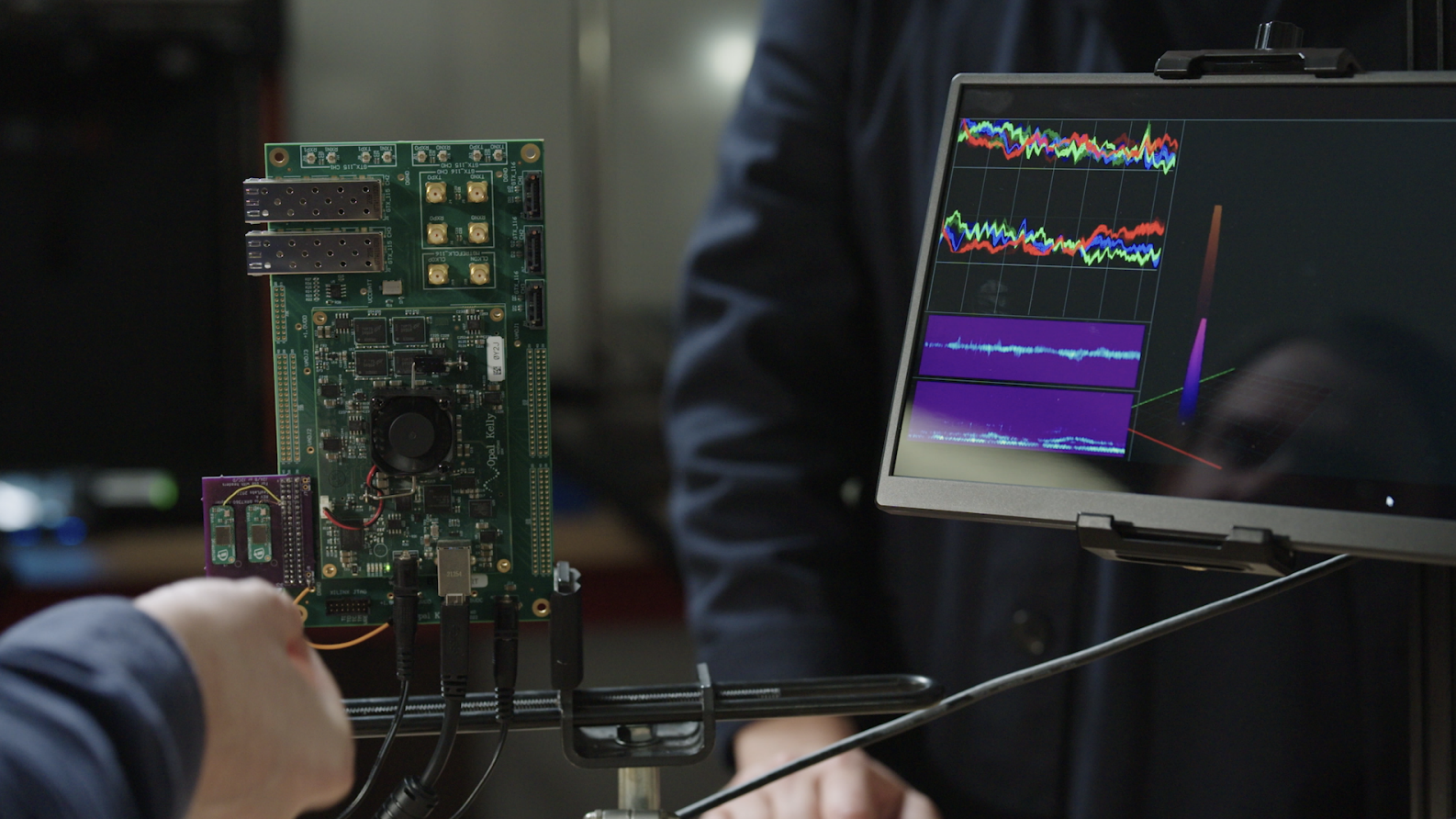
Just as the petabytes of writing on the web underpin LLMs’ human-like reasoning capability, this wealth of real world sensing data can enable physical AI to develop the capability to interpret and reason about the physical world.
But sensor data isn’t text. Incorporating multimodal sensor data into an AI model isn’t trivial because different sensors produce very different raw signals: not just in the format of the data, but also in the information that the data represents. Current ML techniques require a custom processing pipeline or model for each kind of sensor. This approach makes it infeasible to fuse and interpret data across multiple sensing modalities at scale.
Our approach to solve this is to develop a single foundation model for all sensor data, enabled by a universal sensor language.
A Universal Embedding: The “Rosetta Stone” for the Physical World
At their core, LLMs break down language into vectors – mathematical representations of language components, or tokens. These vector representations enable the meaning of any sequence of tokens to be computed by performing mathematical operations on the embedding vectors.
We can do precisely the same with sensor data. By representing the sensor data as a vector in the same embedding space as language, we can compute the meaning of the sensor data using the same mathematical operations.
The key here is the single embedding space that we use for all different sensors, modalities, and language. This universal embedding space allows information to be fused from disparate real world sources and combined with human knowledge captured through language.
Here’s how it works:
- We build foundational encoders for general classes of sensors. The foundation encoders convert raw sensor data into compressed vector representations that retain information about the essential structure of the signal.
- These vectors are then projected into the high-dimensional universal embedding space. Because all the sensor data is now encoded as vectors, patterns in the sensor data are represented as vector relationships in this space.
- Text embeddings are also projected into this space, allowing sensor data to be mapped to semantic concepts.
- Semantic information is then decoded from the universal embedding space into human-understandable formats such as natural language, APIs, or visualizations using multimodal decoders.
The universal embedding space thus acts as a “Rosetta Stone” for deciphering meaning from different kinds of physical world data. It allows natural human interpretation and interaction with physical sensor data.

By mapping disparate sensor data into a single space, it also allows us to use sensors we understand to interpret the ones we don’t. For example, we can capture radar data with camera data and use image labeling on video to label the radar data. Or we can correlate audio recordings from microphones with vibration sensors to monitor and diagnose machinery health, using the sounds and vibrations they emit to predict failures before they happen.
Check out Part 2 of this blog post, where we will explore use cases and practical applications for physical AI in the real world.