As AI's role expands from automating tasks to interpreting our surroundings, developers and users need both a new conceptual framework and practical tools. To meet this need, we're introducing Lenses: a new category of AI applications built on Newton™ that continuously convert raw data into insights tailored to specific use cases. Much like agents or robots are designed for automation, Lenses are designed for interpretation. Read on to learn about a new mental model for Physical AI that simplifies adoption for both developers and users.
From Automation to Augmentation
Since founding Archetype AI in 2023, we've pioneered Physical AI — a concept that received little attention until recently, when NVIDIA CEO Jensen Huang declared it "the next frontier of AI." While current approaches focus primarily on robotics and automating human labor, we're taking a different path: using AI to amplify human intelligence and deepen our understanding of the physical world.
A key part of this vision involves making sense of the massive amounts of sensor data that already exist. Trillions of sensors in factories, buildings, and consumer products continually generate data, but we lack the means to interpret it. At Archetype, we're developing Newton™ — a pioneering AI model that serves as an interpretation layer for the physical world, transforming the overwhelming flood of data from IMUs, cameras, radars, and other types of sensors into intuitive, actionable insights that enhance decision-making. We're not building AI to replace humans, but rather to empower them with deeper understanding of the world around us.
How can we make Physical AI useful to humans and help them solve real-world problems? Throughout 2024, we worked with dozens of customers across industries and discovered that agents don't align well with Physical AI's purpose — they suggest autonomy rather than interpretation.

Instead, we're proposing a new concept called Semantic Lenses, or simply Lenses. These innovative AI applications function like physical lenses, continuously "refracting" raw data from the physical world into actionable insights. Multiple Lenses can be created for different use cases, making Newton™ adaptable to various real-world scenarios. Unlike agents, Lenses don't suggest replacing humans but rather empowering them with AI.
Lenses, the New Metaphor for Physical AI
Metaphors play an important role in shaping our understanding of emerging technologies by bridging the unfamiliar with the familiar. Today, the dominant metaphor for AI is that of an "agent" — manifested as either chatbots or robots. This approach positions AI as an autonomous entity to which we delegate tasks and which then interacts with the world on our behalf.
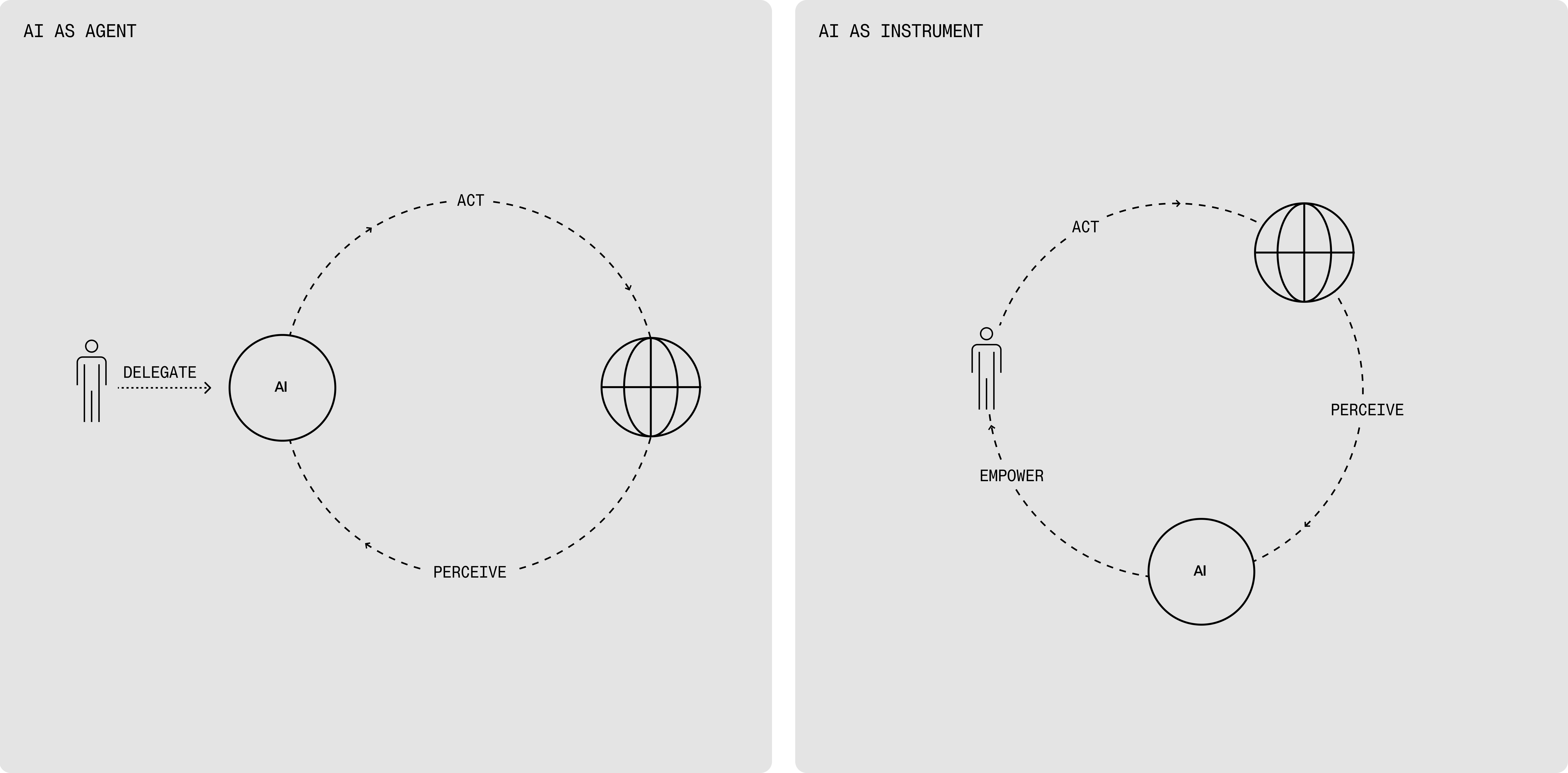
However, when AI serves as an interpretation layer for the physical world, we use it not as an autonomous agent, but as an instrument to probe complex data, keeping the power to act in human hands. As this human-AI relationship shifts from delegation to empowerment, we need a mental model that suggests that AI amplifies, rather than replaces, human decision-making. Inspired by optical lenses, we reimagined how to build and interact with an AI that continuously interprets the world around us.
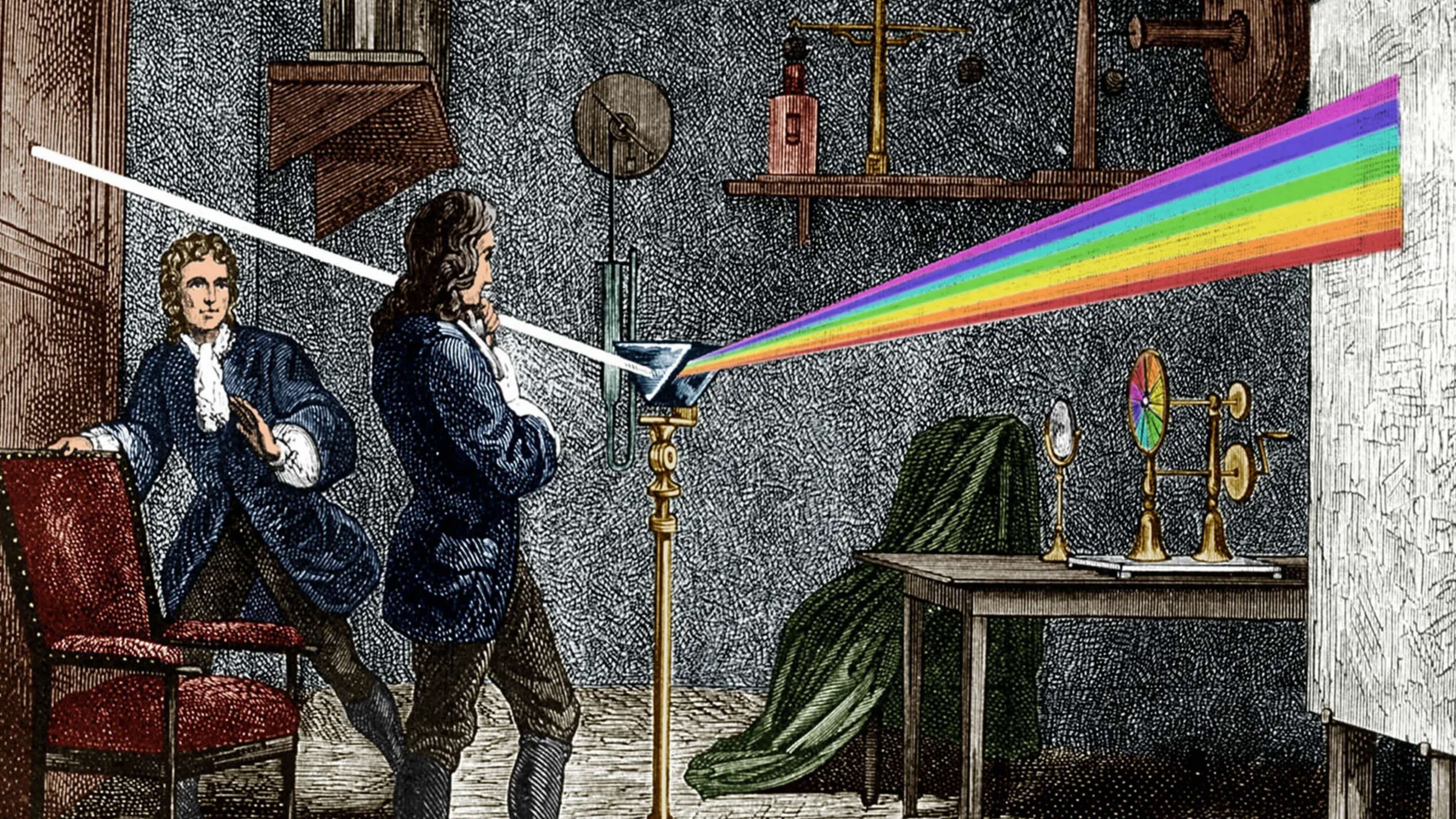
Just as an optical lens transforms incoming light, revealing new information and properties about the world, an AI Lens converts raw data from physical sensors into structured, meaningful information. Unlike agents, which rely on turn-based interactions and explicit user input, AI Lenses operate continuously, adapting to real-world conditions in real time. The Lens metaphor offers a powerful, flexible mental model for designing a new class of AI applications, simplifying adoption by both developers and users.
Lens Customization with Natural Language
Lenses are applications that run on top of Newton™, providing the model with Instructions on how to transform raw sensor data (Input Stream) into a desired interpretation (Output Stream). Because of the alignment between sensor data and language in the Newton model, these Instructions can be expressed in plain English. Among other things, the Instructions could include the operational context of the lens, specify input parameters (sensor modalities, time window), and structure the format of the output.
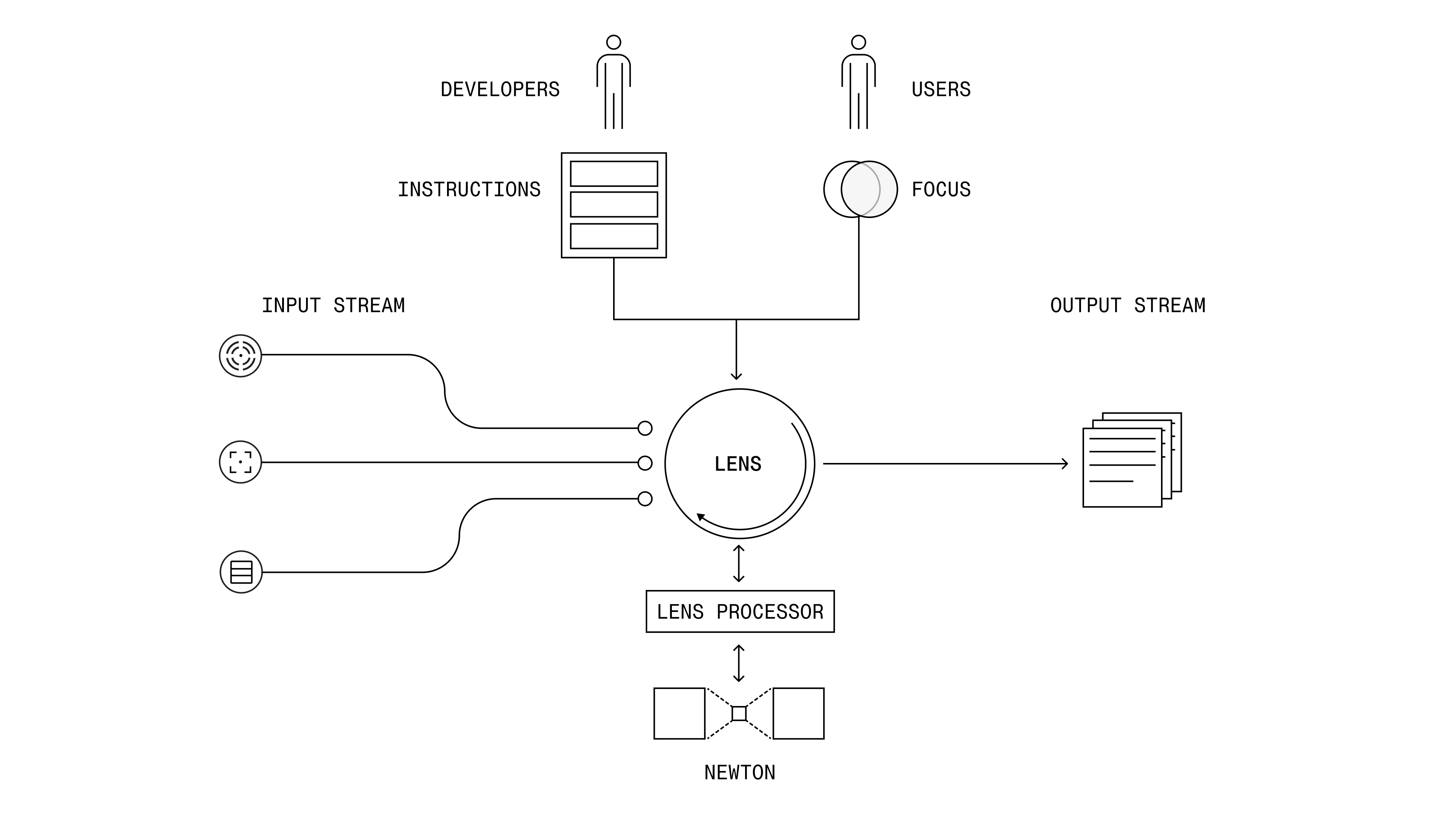
Imagine a Lens designed to interpret traffic data and detect accidents at intersections. This Lens would process camera footage as input, analyzing vehicle and pedestrian behavior to determine whether an incident has occurred. The Instructions could be defined as: "Observe traffic patterns and issue an alert if an accident occurs; otherwise, describe the situation as normal." The output would be a text-based report, using specific tags to highlight incidents. This structured format allows seamless integration into an existing traffic monitoring system, where the message can be displayed directly on the front end, ensuring quick and effective response.
Using the same traffic data, we can design a different Lens with a different operational context and output format. Instead of detecting accidents, this Lens focuses on identifying near-misses (e.g., incidents narrowly avoided) and generates a heat map highlighting high-risk areas at the intersection. City officials monitoring the system could then use this data to make informed decisions on improving road safety.
Focus as Interaction Mechanism
Users can adjust the Focus of an AI Lens at runtime to direct sensor data interpretation toward a desired outcome, while staying within the boundaries set by the Lens Instructions. While the Instructions define the Lens's overall function and behavior, Focus fine-tunes the semantic interpretation to suit the specific task and enable user interaction.
Typically, the Focus is defined using natural language but could include various modalities. In the scenario above, we imagined a Lens running on a pair of sport earbuds, with input from a heartbeat monitor and motion sensors. The Lens is designed to interpret this data and provide recommendations to the runner. The user can adjust the Lens's focus from "improve performance" to "right leg pain," causing it to shift from recommending speed increases to providing injury prevention guidance.
We also built an early demo of the Focus functionality. In this demo, IMU sensor data is used to capture the movement of a package. We designed an AI Lens to interpret this data and provide information about the package's status in real time. The output stream of the Lens changes from events reporting if the package is moving or still, to events describing how the package is handled — e.g., shaken, gently handled, dropped, etc.
Lens Kits
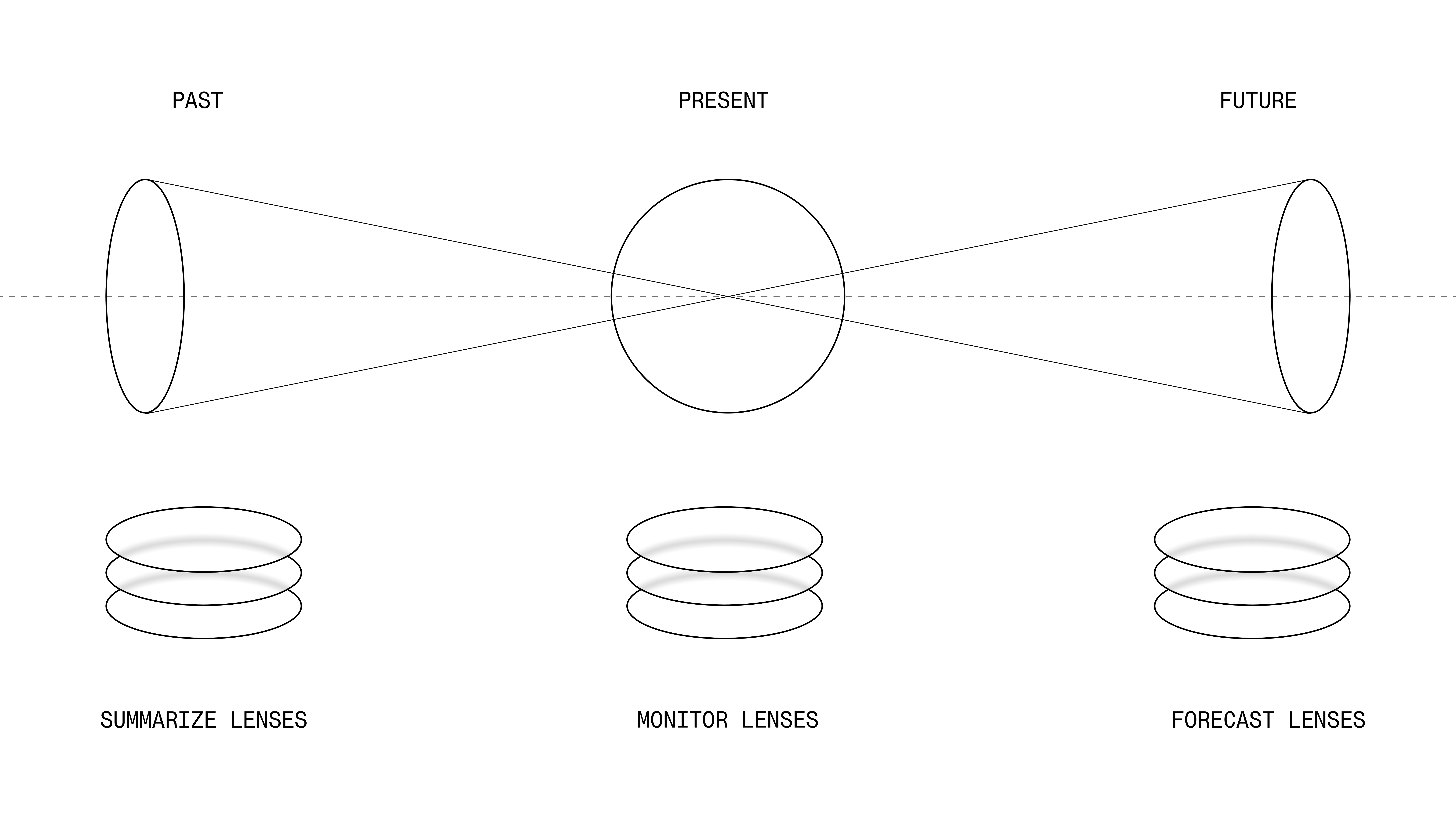
Lenses can generally be grouped into three categories based on the time horizon of the information they analyze: summarizing past data, monitoring present conditions, and forecasting future events.
- Summarize Lenses help users make sense of past data, ranging from a few hours to several years. By adjusting their focus, users can obtain summaries, explanations, and visualizations that improve analysis and decision-making. For example, the "Near-Misses" Lens detects patterns in traffic data to highlight accident-prone areas. Other applications include a Lens that analyzes construction site progress or one that maps space utilization in public buildings to improve escape routes.
- Monitor Lenses enhance human intuition by analyzing sensor data in real time, augmenting perception, and providing timely recommendations. These Lenses work alongside us while we are performing a task. Examples are the "Runner Performance" Lens and the Lens to alert city officials when accidents occur. Additional examples could be a Lens guiding experts in repairing complex machines, providing step-by-step guidance, or a Lens helping safety inspectors quickly identify hazards as they inspect factories, hospitals, or construction sites.
- Forecast Lenses leverage Newton's ability to analyze sensor data trends; they anticipate future events and enhance planning. A key application is predictive maintenance for costly machinery. A Forecast Lens could process wind turbine sensor data to predict failures before they happen, allowing operators to prevent downtime and reduce costs. In healthcare, Forecast Lenses can predict the onset of illnesses and diseases, enabling early intervention and better outcomes.
Lenses provide a practical way to package Newton functionality into an easy-to-use format for both developers and users. Multiple Lenses can be created to address different problems, and users can choose the one best suited for each task.
Toward AI for Intelligence Augmentation
Lenses are a new class of AI instruments for the physical world. Their key benefits include:
- A clear mental model to understand how Physical AI works and what its value is.
- A practical way to package Newton functionality into an easy-to-use format for both developers and users, and seamless integration into existing workflows and systems.
- Customization of Newton's behavior using natural language.
- Focus adjustment at runtime that allows users to direct AI's attention to specific aspects of data.
- Applications across all time horizons: summarizing past data, monitoring present conditions, and forecasting future events.
Just as the prismatic lens revealed hidden structures and transformed scientific discovery, Physical AI doesn't replace human inquiry — it amplifies our capabilities and empowers us to make better decisions in an increasingly complex world.