In a recent blog post, Jakob Nielsen wrote, "ChatGPT and other AI systems are shaping up to launch the third user-interface paradigm in the history of computing—the first new paradigm in more than 60 years." He argues that "with the new AI systems, the user no longer tells the computer what to do. Rather, the user tells the computer what outcome they want."
This new type of interaction is radically changing our relationship with technology and the role it plays in our lives. As designers, we have a critical challenge in front of us: how can we help people make sense of AI? And more specifically, how can we shape AI interfaces to help people build a useful and coherent mental model of this new technology?
Currently, the digital companion is the dominant metaphor we are using to help people understand AI systems. However, as the field of generative AI continues to evolve, it's crucial to examine how we frame and comprehend these technologies. This isn't merely an academic exercise—it will influence how we develop, interact with, and regulate AI. In this blog post, we'll explore different metaphors used in AI products and discuss how they shape our mental models of AI systems.
The Use of Metaphor in AI Systems
Metaphors have been crucial in user interface design since the early days of computing; they act as bridges between familiar and unfamiliar concepts. By mapping known ideas onto new ones, they provide a framework that makes new technology easier to understand. The "desktop" metaphor, for instance, revolutionized how we interact with computers by presenting the digital workspace as a familiar office desk with files, folders, and a trash can.
In his recent TED talk, "What is AI Anyway?," Mustafa Suleyman discusses why metaphors matter when we think about AI — they affect how we build a mental model of AI systems and, therefore, affect how we build and understand it. "We're headed towards the emergence of something that we are all struggling to describe, and yet we cannot control what we don't understand," he explains.
He proposes one way of thinking about AI: digital companions. He explains, "I think AI should best be understood as something like a new digital species. Now, don't take this too literally, but I predict that we'll come to see them as digital companions, new partners in the journeys of all our lives."
Digital companions are currently the dominant metaphor for understanding AI systems. However, as the field of generative AI continues to evolve, it's crucial to examine how we frame and comprehend these technologies. This isn't merely an academic exercise—it will influence how we develop, interact with, and regulate AI.
Companions, Toolkits, and Enchanted Objects
We have investigated a broader set of metaphors that have been used in AI products, beyond the typical ChatGPT-like applications. We have traced their precedents in the history of Human-Computer Interaction, to develop a critical perspective. We have identified three main metaphors: Companion, Toolkit, and Enchanted Object.
Companion
The companion metaphor draws on our understanding of social relationships, centering on human-like interactions that evolve through iterative, multi-turn conversations. This is the most dominant metaphor used in AI products today, exemplified by systems like ChatGPT, Siri, Claude, Dot, Pi, and Alexa.

One of the earliest attempts at a digital companion was ELIZA, a natural language processing system created by Joseph Weizenbaum at MIT in 1966. More recently, voice assistants like Siri or Alexa embody this metaphor, where users converse with the system to achieve their goals.

Throughout human history, technology has often been imagined as companions to humans. From the figure of Golem in Jewish spirituality, a creature made from inanimate matter and brought to life for specific tasks, to HAL 9000 in "2001: A Space Odyssey," we find parallels to modern generative AI—technology controlled by humans through dialogue.
Toolkit
The toolkit metaphor builds on our innate understanding of physical affordances, enabling us to instinctively use and handle tools for specific tasks. Creative software powered by AI often uses this metaphor to quickly familiarize users with AI features: for example, the Magic Eraser in Google Photos, or the Generative Fill in Adobe Photoshop.

There is, of course, a long tradition of interfaces designed using metaphors inspired by tools and physical objects, such as the "desktop" metaphor introduced by Xerox PARC in 1970, or the "toolbox" in MS Paint (1985) that allowed users to create and manipulate images using brushes, buckets, and pickers. Outside the screen, the Marble Answering Machine designed by Bishop in 1992 is a notable example; and more recently, Project Soli (2016) introduced Virtual Tools as a metaphor for gestural interactions.

Historically, Douglas Engelbart was one of the first to talk about the "digital computer as a tool for the personal use of an individual," more specifically as a tool for "augmenting human intellect." This has spawned a tradition in HCI that sees technology as a way of empowering people and amplifying their intelligence rather than replacing it.
Enchanted Object
The Enchanted Object metaphor draws on our ability to conceive objects with properties that transcend their physical nature: see the Magic Mirror in Snow White or the Weasley Clock in Harry Potter. Magic is often used in marketing campaigns to introduce AI products that anticipate and adapt to our needs: "My home is magic" ads by Amazon (2023) show how several products work together to create a safe and comfortable environment.
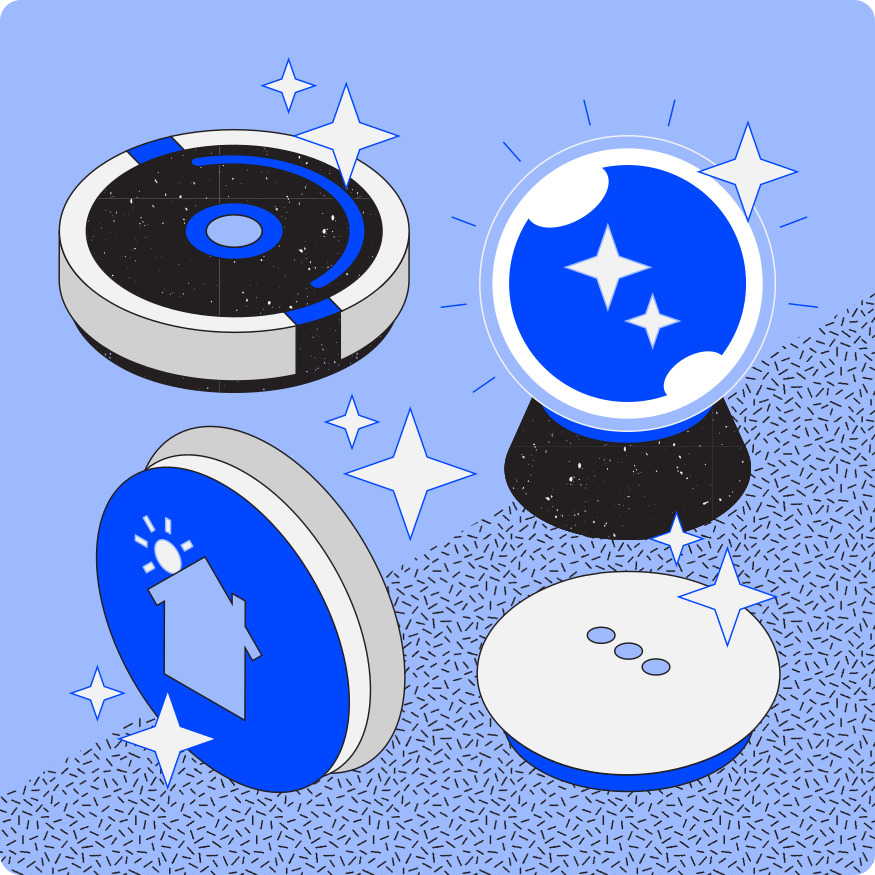
The Enchanted Object metaphor was coined by David Rose in 2015 to describe IoT devices that respond to our needs, behaviors, and desires in intuitive and often magical ways: self-driving cars, smart thermostats, and smart locks are all examples of enchanted objects we encounter in our everyday life.
These devices can all be framed within the vision of Ubiquitous Computing, defined by Mark Weiser in 1996. He imagined a world where technology "disappears" into the things we use everyday, anticipating our needs and delivering relevant information or actions based on context and behavior, without requiring our direct attention.

As shown previously, these metaphors are not new or specific to AI: there are traces of their existence throughout the entire history of HCI. At a fundamental level, these metaphors are grounded in how we, as humans, make sense of the world around us.
In his book "Descartes' Baby," Paul Bloom describes humans as natural-born dualists: as infants, we are immediately able to distinguish between objects and people, and over time we develop distinct theories to describe their behaviors. The Companion metaphor leverages our intuitive understanding of social relationships and the conventions we have created to interact with each other smoothly. Similarly, the Toolkit metaphor takes advantage of our innate ability to understand the physical world, the affordances and behaviors of objects and tools.
Bloom also notices how when we assign mental states to physical things, a new way of understanding the world emerges, such as spiritual explanations or magical thinking. The Enchanted Object metaphor leverages our natural tendency to attribute emotions or mental states to inanimate things as a way to make sense of complex behaviors.
Metaphors as Design Tools
The Companion, Toolkit, and Enchanted Object metaphors provide different frameworks to make sense of AI technologies, taking advantage of our innate ability to understand the world around us, whether physical, social, or magical. More specifically, these metaphors can be used to shape users' mental models of AI systems that are characterized by a different distribution of agency between the user and the AI.
Agency is a fundamental aspect of user-AI interaction. As Nielsen points out, one consequence of how we interact with AI today is that users relinquish control over how tasks are completed. While this simplifies interaction, it has potential downsides: users may struggle to correct errors or guide the system if it misinterprets their intent.
The future of AI systems might blend intent-based interaction with traditional UI controls, allowing users to switch modes based on the task at hand. For example, users could issue broad instructions to an AI while retaining control over fine-tuned adjustments. This creates a hybrid experience where the agency shifts dynamically between user and computer.
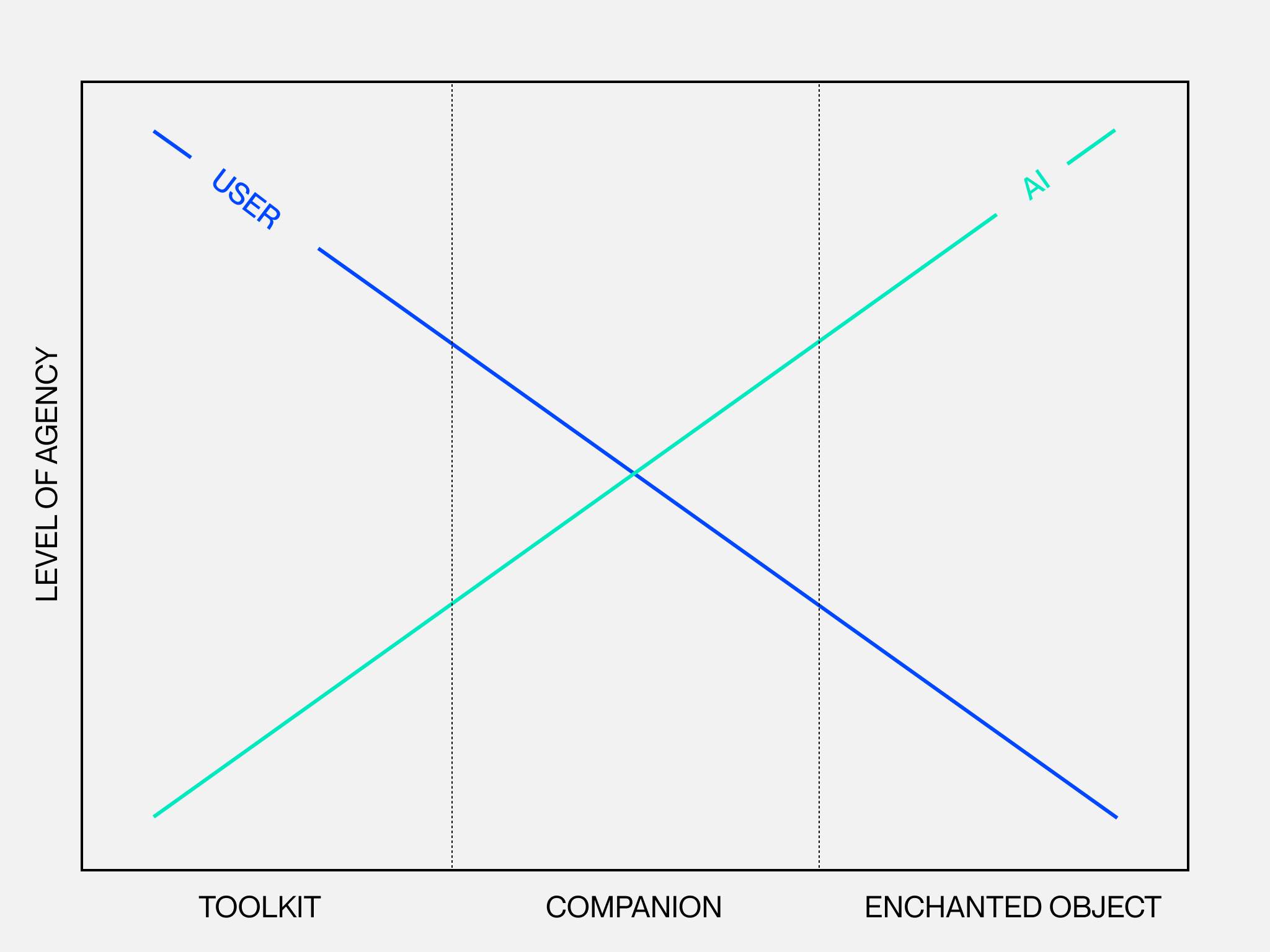
The three metaphors outlined above enable designers to think beyond the status quo and design more sophisticated and useful applications where the agency belongs to the user (Toolkit), shared between the user and the AI (Companion), or controlled by the AI (Enchanted Objects). More specifically:
- The Toolkit metaphor helps design the interaction with AI applications and functionalities for the resolution of specific tasks, under the direct control of the user. This metaphor helps create a mental model where AI is not a monolithic entity but is embodied in a set of specific tools with clear affordances and behaviors that the user can activate for specific tasks.
- The Companion metaphor helps shape a more balanced collaboration between the user and the AI: both parties are engaged in a dialogue, and the outcomes of the task are negotiated. In this case, the AI is often perceived as an entity that not only executes the user's instructions but can creatively contribute to the resolution of the task.
- The Enchanted Object metaphor supports the design of AI applications that operate in the background. The user has little or no control over the outcomes of the interaction: the AI interprets user intentions implicitly and decides the course of action.
Ultimately, choosing the right metaphor depends on the use cases and the users of the product. These metaphors shouldn't be considered in a rigid way. They are resources that designers of AI systems can use to develop a critical perspective, reflect on issues such as control, error correction, transparency, and ultimately shape interfaces that meet people's expectations and mental models. They are not mutually exclusive, and they can be combined to create compelling hybrid experiences.
As AI is transitioning from the digital to the physical world, we're exploring different metaphors and laying the groundwork for defining new interaction models for physical AI. While the status quo borrows directly from the companion metaphor, framing physical AI applications mainly as robotic companions, we believe there's much more potential for Physical AI.
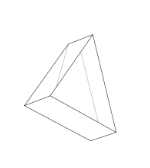